Don’t let your data strategy slow your business down
Published: Jun 25th 2020 | 5 min read
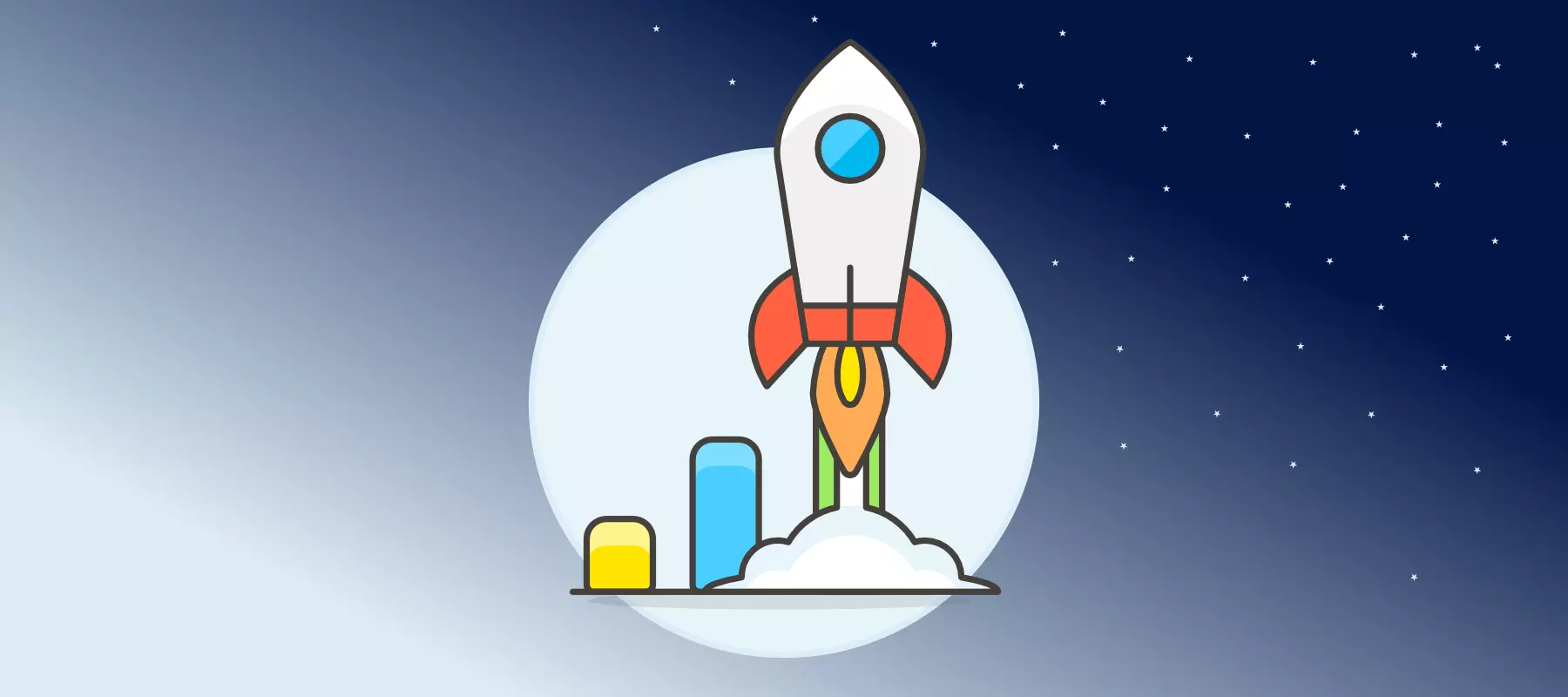
Incorrect decisions can have a damaging impact. Companies gather their resources to combine different data sources, new data streams with existing data, and apply analytics to find connections for better and quicker decisions.
Surveys conducted on the advancement of data maturity show that majority of companies haven’t reached their wished goals despite the excessive investments and numerous attempts. What most companies don’t accept is the amount of effort required to become data-driven and the time progress as well.
Common problems
The most frequent problems businesses face are process efficiency and insufficient improvement of customers’ experience. What can be seen as a first and fastest action to skip the process and suddenly become a data transformation is acquiring the newest and the most expensive technology, without understanding its real purpose, internal process coordination, and company culture. Employees across departments commonly struggle to gain practical insights from available information that are accurate and in time and be able to base their decision on facts, not just estimations.
To be able to extract valuable insights from data, smart investment in the right technology is essential while keeping in mind the data lifecycle. Moving forward, or upward requires the knowledge of the current situation.
Many companies wonder, how to up their game and grow with data. There are various options on how to ensure faster business growth via the right and individually adjusted data strategy. However, the higher and more advanced data strategy, or better known as Data Maturity, needs to be aligned with the business maturity.
Data Maturity
Data Maturity measures how advance is the data analysis and data utilization of the organization. As the companies increase the use of their data, they develop more complex data analytics and processing, the higher stage they achieve.
Maturity should be seen as a long-term goal and is achieved continually, involving many actions. It is iterative and progressive; therefore, companies evolve in smaller projects, which can be broken down into smaller steps, making the progress comprehensible.
Over the years we have cooperated with companies from different stages of the Data Maturity Model, small companies that are starting to combine their data into one stream, while others have integrated advanced machine learning. From the projects we helped to grow, we have acknowledged four stages of data maturity.
Companies that achieved the top level, leverage their increased agility, better partner and supplier cooperations via integration, utilizing data, and predictive analytics. They monetize the data and benefit from significant competitive advantage.
Just naming or defining the phases may not illustrate the true height of the steps, companies have to climb to score the next stage. The gap is bigger than it seems.
1. Data Novice
In the first stage, the company initializes the data journey with manual non-standardized reporting in different systems, different data sources. The firm recognizes the need for collecting data without building a data structure or systematic analysis. The reports are often irregular, and the business does not rely on its data. Usually, SME and startups are in this phase.
2. Data Standardized
Data storage is incomplete, and the company uses multiple databases, and the biggest question is data quality. The company is ready to start data initiative and is looking for know-how on how to manipulate or use the data. What can be seen here is that IT hits a wall of capacity and capability to advance the data strategy.
3. Data Advanced
Critical decisions are based on data. Data is being broken down throughout the entire organization and it is used as a competitive differentiator. Executive engagement is needed for reaching a higher level. Optimization of data storage and platforms is required to keep up with the demand.
4. Data-Driven
A phase characterized as no data - no decision. Technologies and business reached tight cooperation and are fully integrated. The company has been able to identify the processes for data analytics implementation and it became part of every company process, reaching prescriptive analytics. The speed of interactions in development increases. The data infrastructure is secure, yet it needs to be able to recognize the attacks and reacts to all the changes in real-time.
Comparison of each stage by characteristics and focus
For a transformation to becoming a data-driven organization, true differentiation lays in a strategic decision of leadership and treating the company’s data as a strategic asset. From the data collection throughout the whole data flow and architecture, up to its visualization, exploring new opportunities of modern and adequate technology separates the market leaders from the followers.
Conclusion
Rising to a higher stage of data maturity takes a greater amount of resources, especially time and investment in technology and talents. With the growing amount of data produced each day, the returns of the investment come sooner in the form of more loyal customers, higher conversions, cost reduction, and much more.
Get to know our solutions: